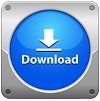
According to Basel 2, each bank needs to organize and develop its own internal credit scoring system with which they can analyze a borrower’s risk. Customers with a high probability of loan repayment are classified in the good customer group and customers with a high probability of default are classified in the bad customer group (Akkoc, 2012).Ĭredit risk assessment is vital for banks they must ensure that borrowers are able to pay their installments before allocating a loan to them (Narindra Mandalaa & Fransiscus, 2012). It is defined as the probability of non-payment or delayed payment by customers or their inability to repay a loan (Cisko & Klieštik, 2013). According to the Basel 2 accord, credit risk is one of the risks that banks face in allocating resources. Therefore, when banks are faced with a risk, appropriate risk management depends on identifying, understanding, measuring, and finally providing appropriate strategies towards it (Bekhet & Eletter, 2014). This is delicate because a bank’s survival is tied to taking appropriate risks a non-risk-taking bank is as vulnerable as an overly-risk-taking one (Narindra Mandalaa & Fransiscus, 2012). Inevitably, banks must take risks in giving loans and credit cards to customers because these are economic drivers. We assert that this model is a good substitute for the static models currently in use as it can outperform traditional models, especially in the face of economic crisis.Ī commercial bank, hereafter referred to as a bank, is a type of financial institution that provides services such as accepting deposits, making business loans, and offering basic investment products. The latest created model is used for assessing customers henceforth, so the whole process of customer assessment need not be repeated. The major innovation of this research is producing a table of bad customers on a monthly basis and creating a dynamic model based on the table.
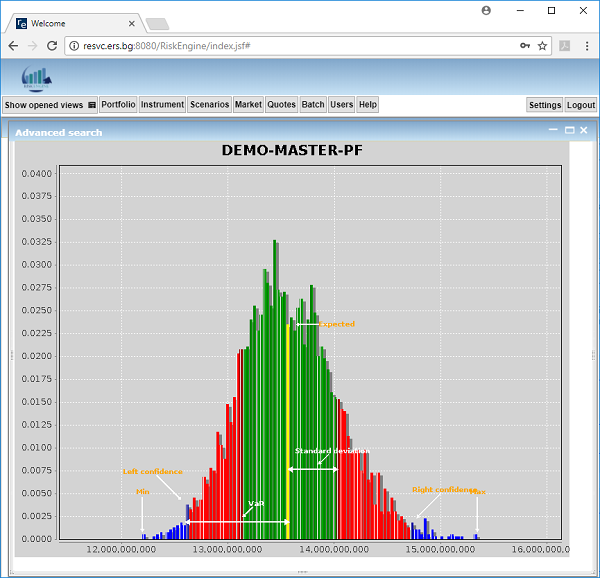
Credit risk specialists also approve the results.
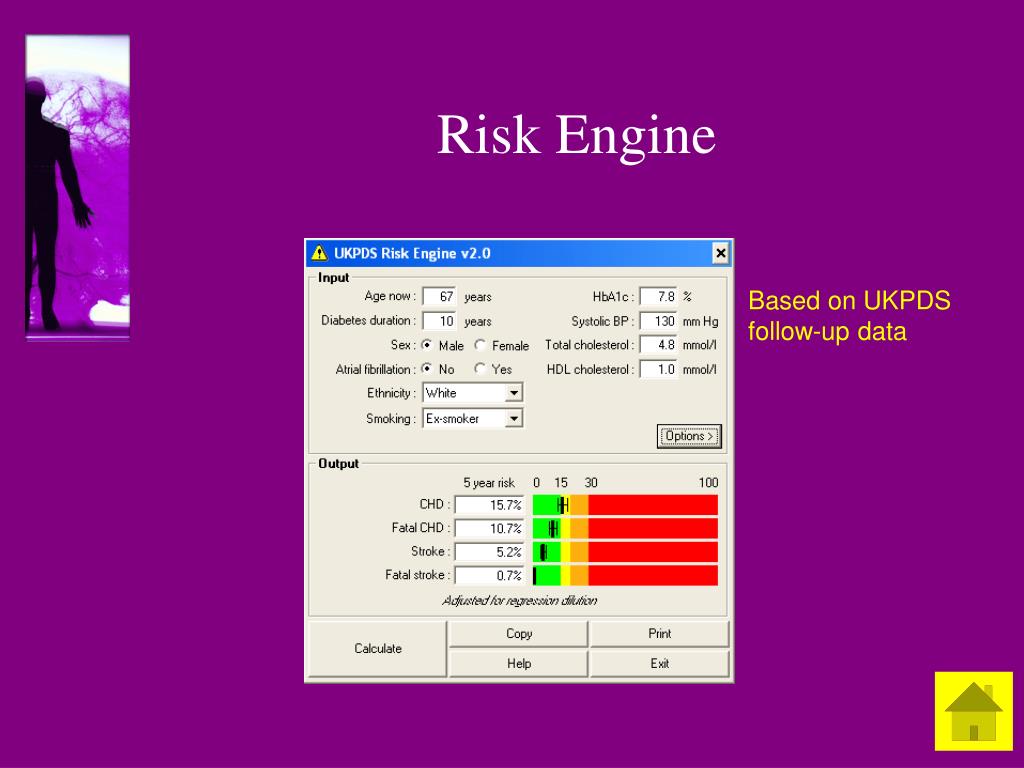
Comparison between the prediction made by proposed model and a real non-performing loan indicates little difference between them. Thus, we present a model that is both more flexible to politico-economic factors and can yield results that are max compatible with real-life situations. Then, using the newly defined factors and their underlying rules, a second round of assessment begins in a fuzzy inference system. First, we train an adaptive network-based fuzzy inference system (ANFIS) using monthly data from a customer profile dataset. We used a fuzzy inference system to create a rule base using a set of uncertainty predictors. Human judgement is removed from the customer evaluation process. In this paper, we propose a model that can accommodate factors associated with politico-economic crises. Nevertheless, a dynamic model that can accommodate fluctuating politico-economic factors has never been developed. This has been especially evident in Iran after the 2008–2016 USA sanctions, as many previously reliable customers became unable to repay their debt (i.e., became bad customers). However, economic factors are not independent of political fluctuations, and as the political environment changes, the economic environment evolves with it. Traditionally, banks have used static models with demographic or static factors to model credit risk patterns. Some banks have such systems nevertheless they have lost a large amount of money simply because the models they used failed to accurately predict customers’ defaults. According to the Basel 2 guidelines, banks need to develop their own credit risk assessment systems.

Giving loans and issuing credit cards are two of the main concerns of banks in that they include the risks of non-payment.
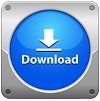